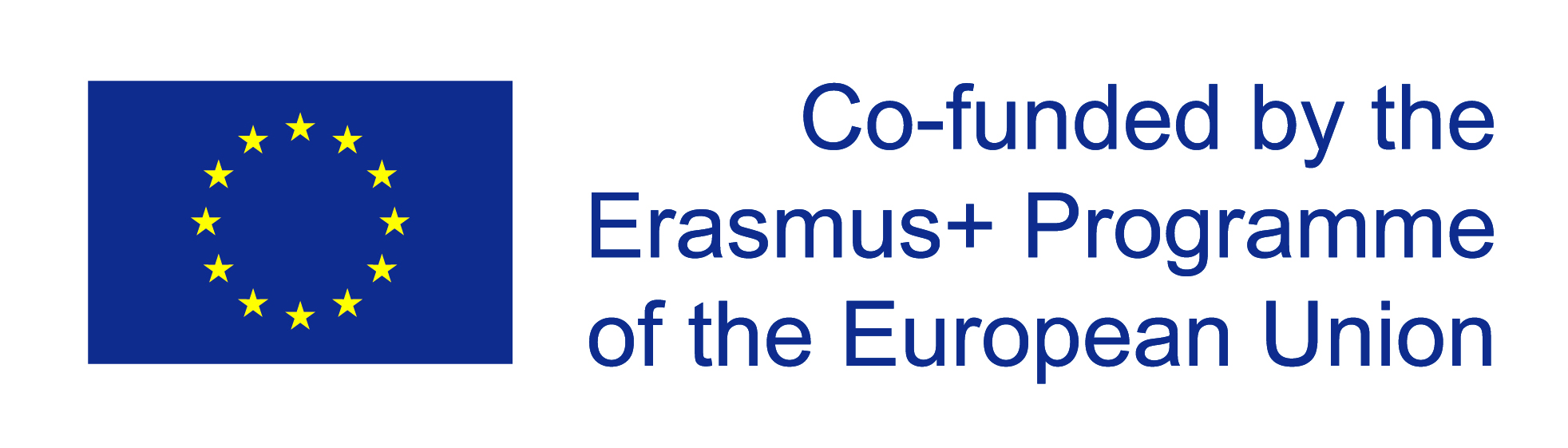
This project (2020-1-SE01-KA203-077872) has been funded with support from the European Commission. This web site reflects the views only of the author, and the Commission cannot be held responsible for any use which may be made of the information contained therein.